일 | 월 | 화 | 수 | 목 | 금 | 토 |
---|---|---|---|---|---|---|
1 | 2 | 3 | 4 | 5 | ||
6 | 7 | 8 | 9 | 10 | 11 | 12 |
13 | 14 | 15 | 16 | 17 | 18 | 19 |
20 | 21 | 22 | 23 | 24 | 25 | 26 |
27 | 28 | 29 | 30 |
- dinov2: learning robust visual features without supervision
- vlm hallucination paper
- clip adapter
- 원격 학습 안끊기게
- dinov2: learning robust visual features without supervision 논문 리뷰
- 1차 미분 마스크
- dinov2 논문 리뷰
- evaluating object hallucination in large vision-language models 논문
- vlm
- 엔트로피란
- clip
- dinov2: learning robust visual features without supervision 논문
- evaluating object hallucination in large vision-language models
- 기계학습
- 논문 요약
- 이미지 필터링
- 에지 검출
- blip-2
- object detection
- 딥러닝 엔트로피
- vlm 환각
- vlm hallucination
- 객체 검출
- 논문 리뷰
- mobilenetv1
- Object detection article
- 딥러닝 목적함수
- vlm 환각이란
- evaluating object hallucination in large vision-language models paper
- polling-based object probing evaluation
- Today
- Total
My Vision, Computer Vision
[논문 리뷰/요약] EfficientVLM: Fast and Accurate Vision-Language Models via Knowledge Distillation and Modal-adaptive Pruning 본문
[논문 리뷰/요약] EfficientVLM: Fast and Accurate Vision-Language Models via Knowledge Distillation and Modal-adaptive Pruning
gyuilLim 2025. 3. 31. 14:25EfficientVLM: Fast and Accurate Vision-Language Models via Knowledge Distillation and Modal-adaptive Pruning
Pre-trained vision-language models (VLMs) have achieved impressive results in a range of vision-language tasks. However, popular VLMs usually consist of hundreds of millions of parameters which brings challenges for fine-tuning and deployment in real-world
arxiv.org
Author : Wang, Tiannan, et al.
Journal : Arxiv
Keyword : Knowledge distillation
Published Date : 2022년 10월 14일
Problem
- 본 연구에서는 Distilling then pruning 프레임워크를 사용하여 Large Vision-Language model을 더 작고, 빠르고, 정확하게 만든다.
- NLP, Vision-Langauge 모두 트랜스포머 기반 사전학습 모델이기 때문에 수 억개, 수십 억개의 파라미터를 가지고있다.
- LLM(BERT 등)의 Knowledge Distillation은 많이 연구되지만 VLM에 대한 선행 연구는 부족하다.
- 기존 연구(Distill VLM등)는 Object-Feature based VLM에 국한된다.
- 따라서 Compact VLM은 기존 일반 VLM에 비해 성능이 부족하다.
Methods
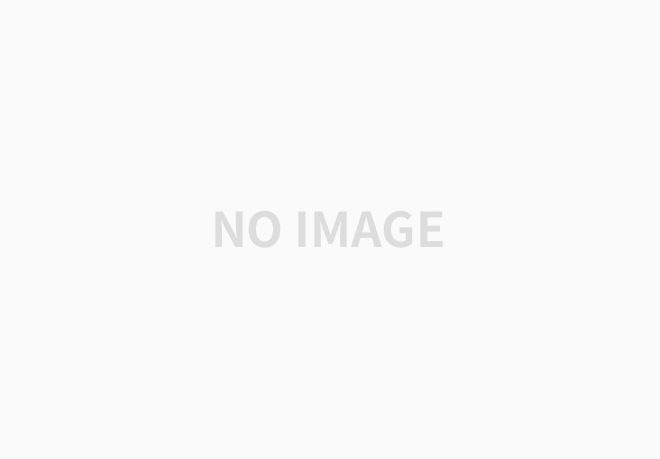
Model Overview
- 당시 SOTA 모델 중 하나인 X-VLM을 Teacher 모델로 사용한다.
- EfficientVLM은 X-VLM의 압축된 버전으로, Transformer-based VLM이다.
- X-VLM은 ALBEF와 똑같이 Image Encoder, Text Encoder, Cross-Modal Encoder로 구성되며, 각각 Transformer layer는 12, 6, 6개이다.
- EfficientVLM은 X-VLM의 크기를 절반으로 줄인 모델(6, 3, 3개)이다.
- 또한 Teacher Model(X-VLM)은 Multi-granularity 방식으로 Text, Visual Concepts를 정렬한다.
LVLP=LITC+LITM+LMLM+LBBOX
Pre-training with Knowledge Distillation
- EfficientVLM을 Pre-trained X-VLM으로 초기화한 후 짝수 번호의 레이어만 남겨서 크기를 절반으로 줄인다.
- 그 후 Image-Text pair로 훈련하는데, X-VLM의 원래 Objective와 Pre-trained X-VLM을 Teacher 모델로 사용하는 Knowledge Distillation Objective 모두 사용한다.
- Knowledge Distillation Objective는 Attention Distillation, Hidden states Distillation, Logits Distillation으로 구성된다.
Attention Distillation
- Self-attention matrices는 아래와 같다.
A=softmax(Q⋅K/√dk)
- Attention Dstillation loss는 Techer와 Student 모델 간 Attention matrices의 MSE로 계산된다.
Lattn=1hL∑j=1h∑i=1MSE(ASi,j,ATi,2j)
- L은 Layer 개수, h는 Attention head 개수를 의미한다.
Hidden States Distillation
- TinyBERT처럼, Techer 모델의 정보를 더 잘 활용하기 위해 Hidden States Distillation을 채택한다.
- 손실 함수는 아래와 같이 정의된다.
Lhid=L∑i=1MSE(HSi,HT2i)
Logits Distillation
- 모델의 출력값인 Logits의 분포를 맞추기 위한 방법이다.
- KL divergence를 손실 함수로 사용해 Teacher, Student의 Logits의 분포를 가깝게 맞춘다.
Pre-training
- 따라서 최종 Loss는 원래의 VLM Objective와 Distillation Objective를 합하여 계산한다.
LKD=αLattn+βLhid+γLlogits Lpretrain=λLVLP+(1−λ)LKD
- α,β,γ,λ는 Loss의 Weight이다.
Fine-tuning with Pruning
- 하나의 Transformer Encoder만 존재하는 BERT와는 다르게 VLM은 구성 모듈들의 중요도가 같지 않다.
- 따라서 이를 증명하기 위해 Image-Text Retrieval, NLVR(Natural Language Vision Reasoning)에서 Pruning 에 따른 성능 하락을 비교한다.
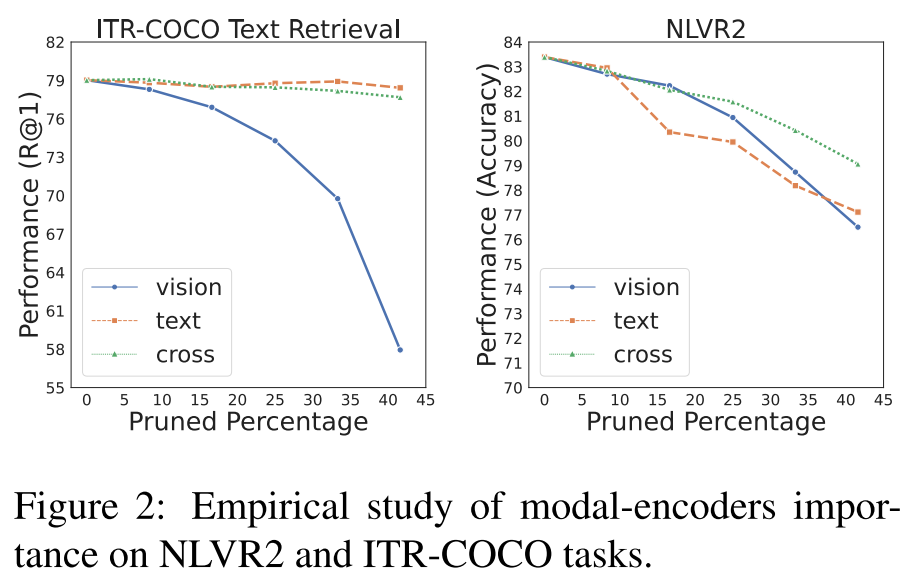
- 먼저 ITR-COCO Text Retireval에서는 Vision Encoder가 다른 모듈들에 비해 프루닝에 대한 성능이 민감하다.
- NLVR2에 대해서는 Vision Encoder, Text Encoder에서는 두 모듈 다 중요하며, Cross Modal 모듈은 비교적 중요도가 낮다고 해석할 수 있다.
Model Adaptive Pruning
- 단순한 접근을 위해, Vision Encoder, Text Encoder, Cross-Modal Encoder 모듈의 각 Pruning 비율을 30%로 설정한 모델을 Baseline을 설정하고 실험을 진행한다.
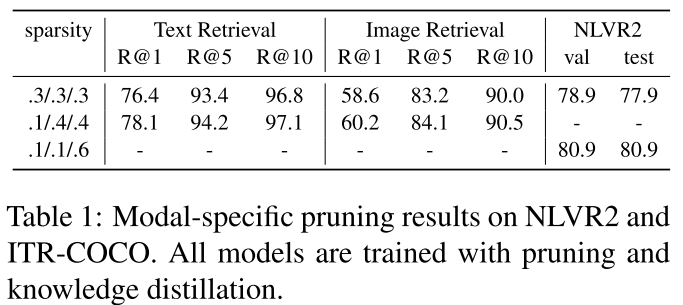
- Text Retireval과 Image Retreival에서는 Vision Encoder의 Pruning 비율을 줄이고, Text Encoder, Cross-Modal Encoder의 Pruning 비율을 높인 것이 효과가 더 좋다.
- NLVR2에서는 VIsion Encoder, Text Encoder의 Pruning 비율을 낮추고 Cross-Modal Encoder의 Pruning 비율을 높인 것이 효과가 더 좋다.
- 위 결과로 Modal-specific Pruning의 효과를 입증할 수 있다.
- 하지만 Task 별 Pruning 비율을 수동으로 설정하는 것은 비용이 많이들고 최적이지 않을 수 있다.
- 따라서 Modal-Adaptive Pruning을 제안한다. 이 방법은 Vision, Langauge Modal의 중요도를 추론하고, 각 인코더에서 중복된 구주와 뉴런을 제거한다.
- 이제 실제로 Pruning은 어떻게 수행되는지 알아보자.
- 먼저, 파라미터 집합 \boldsymbol {\theta} = {\theta}^n_{j=1} 로 이루어진 모델 f(\cdot; \boldsymbol \theta)가 있다. 각 \theta_j는 보통 Block 단위 Weight를 의미한다.
- 이제 새로운 이진 변수 \mathbf z = { z_j }^n_{j=1}를 도입하여 Pruning을 결정한다. 학습할 때 \mathbf z는 0과 1사이의 범위로 설정되고, 추론 단계에서 특정 임계값 이하면 0, 이상이면 1로 설정되어 0인 부분은 사라지게되어 Pruning이 되는 것이다.
\hat{\boldsymbol \theta} = \boldsymbol \theta \odot \mathbf z \;\;\;\; \forall j \; \; \hat \theta_j = \theta_j z_j
- Pruning 후 남겨진 파라미터 집합을 \hat{\boldsymbol \theta}라고 한다. 또한 ||\hat {\boldsymbol \theta}||0 = \sum^n{j=1}z_j로, 남겨진 Block의 개수를 의미한다.
- 따라서 Model Adaptive Pruning의 학습은 아래의 Objective를 최소화하는 방향으로 학습된다.
\hat{\boldsymbol \theta} = \boldsymbol \theta \odot \mathbf z \; \; \; \; \forall j \; \; \hat \theta_j = \theta_j z_j
- 또한 Knowledge Distillation도 태스크 별 파인튜닝을 진행한다. 최종적인 Loss는 아래와 같다.
\mathcal L_{ft} = \lambda \mathcal L_{VL} + (1-\lambda) \mathcal L_{KD} + \mathcal L_{Lgr}
- \mathcal L_{VL}은 태스크 별 Fine-tuning 될 때의 손실, \mathcal L_{KD}는 태스크 별 Knowledge Distillation 손실, \mathcal L_{Lgr}은 Pruning 관련 라그랑주 손실을 의미한다.