반응형
250x250
Notice
Recent Posts
Recent Comments
Link
일 | 월 | 화 | 수 | 목 | 금 | 토 |
---|---|---|---|---|---|---|
1 | 2 | 3 | 4 | 5 | ||
6 | 7 | 8 | 9 | 10 | 11 | 12 |
13 | 14 | 15 | 16 | 17 | 18 | 19 |
20 | 21 | 22 | 23 | 24 | 25 | 26 |
27 | 28 | 29 | 30 |
Tags
- evaluating object hallucination in large vision-language models paper
- dinov2 논문 리뷰
- blip-2
- Object detection article
- evaluating object hallucination in large vision-language models 논문
- 객체 검출
- 논문 리뷰
- object detection
- vlm hallucination
- mobilenetv1
- dinov2: learning robust visual features without supervision
- dinov2: learning robust visual features without supervision 논문
- vlm 환각이란
- vlm hallucination paper
- vlm
- dinov2: learning robust visual features without supervision 논문 리뷰
- 딥러닝 목적함수
- 원격 학습 안끊기게
- clip adapter
- 에지 검출
- 논문 요약
- 1차 미분 마스크
- 기계학습
- polling-based object probing evaluation
- 딥러닝 엔트로피
- clip
- 이미지 필터링
- evaluating object hallucination in large vision-language models
- vlm 환각
- 엔트로피란
Archives
- Today
- Total
My Vision, Computer Vision
[논문 리뷰/요약] An Image is Worth 16x16 Words: Transformers for Image Recognition at Scale 본문
Paper
[논문 리뷰/요약] An Image is Worth 16x16 Words: Transformers for Image Recognition at Scale
gyuilLim 2024. 8. 27. 19:38
An Image is Worth 16x16 Words: Transformers for Image Recognition at Scale
While the Transformer architecture has become the de-facto standard for natural language processing tasks, its applications to computer vision remain limited. In vision, attention is either applied in conjunction with convolutional networks, or used to rep
arxiv.org
Abstract
- Transformer가 사실상 NLP 분야의 표준이 되었지만 Computer vision에 적용하는 데에는 여전히 한계가 있다.
- Computer vision에서 Attention 기법은 CNN과 함께 사용되기도 하고 일부 Layer를 대체하기도 하는데, 본 논문에서는 CNN에 의존하지 않고 Transfomer만 적용한 방법을 제시한다.
Introduction
- Transfomer를 이미지에 바로 적용하기 위해, 우선 이미지를 패치로 나눈 후 Linear Embedding을 거친다.
- 이 때 각 패치는 위치에 따른 Sequence Data로 취급된다. 즉, 이미지 패치가 NLP에서의 Token의 역할을 한다.
- Transfomer는 CNN과 비교했을 때 Inductive bias가 낮다.(= 일반화 능력이 떨어진다.) 따라서 대량의 데이터셋에서 Pretrain을 거친 후 Downstream task에 적용해야 높은 성능을 기대할 수 있다.
Related Work
- 기존 연구를 살펴봤을 때, Self-attention을 이미지에 적용하는 Naive한 방법은, pixel 단위로 연산하는 것이다.
- 하지만 입력 이미지의 모든 픽셀값을 연산에 사용하면 비용이 커지기 때문에 Approximation(근사)을 적용한다.(ex. 이미지 패치)
Method
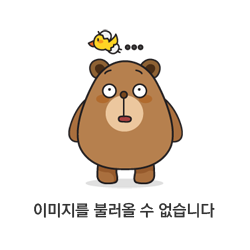
- VIT의 전체적인 구조를 나타낸 사진이다.
- 입력 이미지를 패치로 나눈 후, 각각을 Linear Projection을 통해 벡터로 변환한다.
- 변환된 벡터가 Transfomer Encoder를 거친 후, MLP Head에서 연산 후 분류 결과를 출력한다.
Patch Embedding
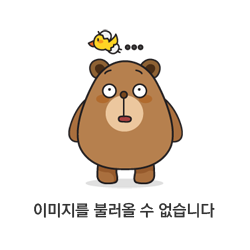
- VIT 구조에서 위 단계가 Patch Embedding이다.
- Transformer는 입력으로 1차원 벡터를 받기 때문에 이미지를 1차원으로 Flatten해야하는데, 이것을 Patch Embedding이라고 한다.
- 먼저
크기의 이미지를 개의 패치로 분할( ) 한다. : Height, Width, Channel : Number of Patch : Patch size
- 그 후 Linear Projection(Fully Connected Layer)을 거쳐
크기의 차원으로 매핑한다. - 따라서 Patch Embedding 후 Output의 크기는
가 된다.
# Patch Embedding
patch_embedding = nn.Sequential(
Rearrange('b c (h p1) (w p2) -> b (h w) (p1 p2 c)', p1 = patch_height, p2 = patch_width),
nn.LayerNorm(patch_dim),
nn.Linear(patch_dim, dim),
nn.LayerNorm(dim),
)
- VIT 코드를 살펴보면 Patch Embedding은 위와 같이 구현되어있다.
- Rearrange가 Reshape & Flatten 부분인데, 예를들어 3 * 30 * 30 크기의 이미지가 4 배치로 묶여있고 패치의 높이, 너비가 각각 5라고 했을 때 4 * 3 * 30 * 30 → 4 * 3 * (6 * 5) * (6 * 5) → 4 * (6 * 6) * (5 * 5 * 3) →
4(B) * 36(N) * 75(patch_dim) 가 된다. - 즉 하나의 3 * 30 * 30 이미지를 5 * 5 크기의 패치로 나누면 1채널당 5 * 5 크기의 패치가 총 36(=6 * 6)개 만들어지기 때문에 36(=6*6) * 75(= 3 * 25 * 25)가 되는 것이다.
- 이어서 Linear 연산을 통해 patch_dim(ex. 75)를 dim으로 매핑한다.
Position Embedding
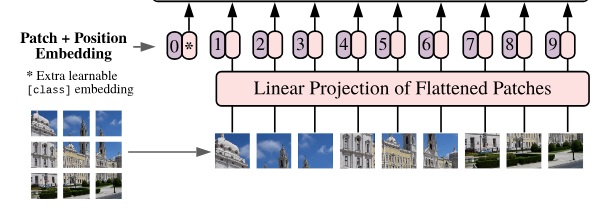
- 위 사진처럼 입력 이미지에서 총 9개의 패치가 만들어진다고 했을 때, 0번째 패치부터 8번째 패치까지 만들어진다.
- 이 때 각각의 Index에 대한 Embedding값을 Linear Projection으로부터 계산된 10개(+ extra)의 벡터에 각각 더해준다.
- 더해지는 Position Embedding 값은 Trainable한 값들이다.
# Position Embedding
pos_embedding = nn.Parameter(torch.randn(1, num_patches + 1, dim))
x = x + self.pos_embedding[:, :(n + 1)]
Transformer Encoder
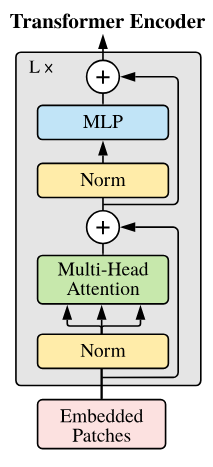
- VIT의 Transfomer Encoder는 기존 구조와 유사하다. Attention Block과 Feed Forward Block으로 구성된다.
- Encoder stack은 L로 설정한다.(L = 12, 24, 32)
- Transfomer Encoder의 연산 과정을 수식으로 나타내면 다음과 같다.
- (1), Embedded Patches : Transformer Encoder의 Initial Input을 의미한다.
개의 패치 각각에 임베딩 행렬 를 곱하고 Position Embedding 를 곱해준 것이다. - (2), Attention Block : LN은 Layer Norm, MSA는 Multihead self-attention을 의미한다. 이전 Encoder layer의 Input을 받아, LN과 MSA 연산 후 Residual connection(
)까지 더해준다. - (3), Feed Forward Block : (2)에서 연산된 결과를 입력으로 받아 LN, MLP을 차례대로 거친다. 이 때도 마찬가지로 Residual Connection을 더해준다.
- (4), Transfomer Encoder Output : (3)의 결과를 마지막으로 LN하면 최종 출력인
가 만들어진다.
# Transformer Encoder
class Transformer(nn.Module):
def __init__(self, dim, depth, heads, dim_head, mlp_dim, dropout = 0.):
super().__init__()
self.norm = nn.LayerNorm(dim)
self.layers = nn.ModuleList([])
for _ in range(depth):
self.layers.append(nn.ModuleList([
Attention(dim, heads = heads, dim_head = dim_head, dropout = dropout),
FeedForward(dim, mlp_dim, dropout = dropout)
]))
def forward(self, x):
for attn, ff in self.layers:
x = attn(x) + x
x = ff(x) + x
return self.norm(x)
- 설정된 depth 만큼 Attention block과 Feed Forward block을 반복하는 구조로 만들어진다.
728x90