일 | 월 | 화 | 수 | 목 | 금 | 토 |
---|---|---|---|---|---|---|
1 | 2 | 3 | ||||
4 | 5 | 6 | 7 | 8 | 9 | 10 |
11 | 12 | 13 | 14 | 15 | 16 | 17 |
18 | 19 | 20 | 21 | 22 | 23 | 24 |
25 | 26 | 27 | 28 | 29 | 30 | 31 |
- 구글 서머 오브 코드 합격 후기
- 기계학습
- gsoc 후기
- albert 논문 리뷰
- 논문 리뷰
- 이미지 필터링
- 딥러닝 엔트로피
- 구글 서머 오브 코드
- 딥러닝 목적함수
- mobilenetv1
- albert: a lite bert for self-supervised learning of language representations
- object detection
- 1차 미분 마스크
- gsoc 2025
- gsoc 합격 후기
- 구글 서머 오브 코드 후기
- 엔트로피란
- gsoc 2025 후기
- blip-2
- albert 논문
- 논문 요약
- 에지 검출
- albert paper
- albert 모델
- clip adapter
- Object detection article
- 객체 검출
- vlm
- clip
- 원격 학습 안끊기게
- Today
- Total
목록분류 전체보기 (78)
My Vision, Computer Vision
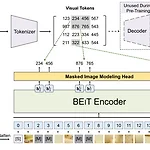
BEiT: BERT Pre-Training of Image TransformersWe introduce a self-supervised vision representation model BEiT, which stands for Bidirectional Encoder representation from Image Transformers. Following BERT developed in the natural language processing area, we propose a masked image modeling task to prearxiv.orgProblemBEIT(Bidirectional Encoder representation from Image Transformers)를 제안한다.ViT는 CNN..
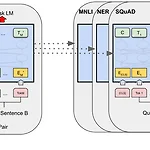
BERT: Pre-training of Deep Bidirectional Transformers for Language UnderstandingWe introduce a new language representation model called BERT, which stands for Bidirectional Encoder Representations from Transformers. Unlike recent language representation models, BERT is designed to pre-train deep bidirectional representations from unlaarxiv.org발행일 : 2018. 10. 11.Google AI LanguageProblem기존의 Langu..
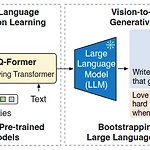
BLIP-2: Bootstrapping Language-Image Pre-training with Frozen Image Encoders and Large Language ModelsThe cost of vision-and-language pre-training has become increasingly prohibitive due to end-to-end training of large-scale models. This paper proposes BLIP-2, a generic and efficient pre-training strategy that bootstraps vision-language pre-training from oarxiv.org Problem대부분의 State-of-the-art V..
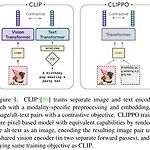
CLIPPO: Image-and-Language Understanding from Pixels OnlyMultimodal models are becoming increasingly effective, in part due to unified components, such as the Transformer architecture. However, multimodal models still often consist of many task- and modality-specific pieces and training procedures. For example,arxiv.orgProblem대부분의 멀티모달 모델은 모달리티 별로 구성 요소가 다르고, 다른 태스크에 적용하기 위해 그에 맞는 추가적인 학습 절차가 필요..
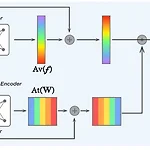
CLIP-Adapter: Better Vision-Language Models with Feature AdaptersLarge-scale contrastive vision-language pre-training has shown significant progress in visual representation learning. Unlike traditional visual systems trained by a fixed set of discrete labels, a new paradigm was introduced in \cite{radford2021learning}arxiv.orgProblem(문제 지적)CLIP은 클래스 이름을 프롬프트 템플릿에 넣어 Zero-shot transfer를 수행하는데, 이..
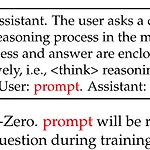
DeepSeek-R1: Incentivizing Reasoning Capability in LLMs via Reinforcement LearningWe introduce our first-generation reasoning models, DeepSeek-R1-Zero and DeepSeek-R1. DeepSeek-R1-Zero, a model trained via large-scale reinforcement learning (RL) without supervised fine-tuning (SFT) as a preliminary step, demonstrates remarkable reasoninarxiv.orgAbstract본 논문에서는 DeepSeek-R1-Zero와 DeepSeek-R1이라는 모델..